概要
今回は、Transformerについて、モデルアーキテクチャとソースコードとを比較しながら解説を行います。前回は概念的な説明でしたが、ソースコードとあわせて見ることで、より理解が深まると思います。今回解説をするソースコードはこちらになります。
Transformer
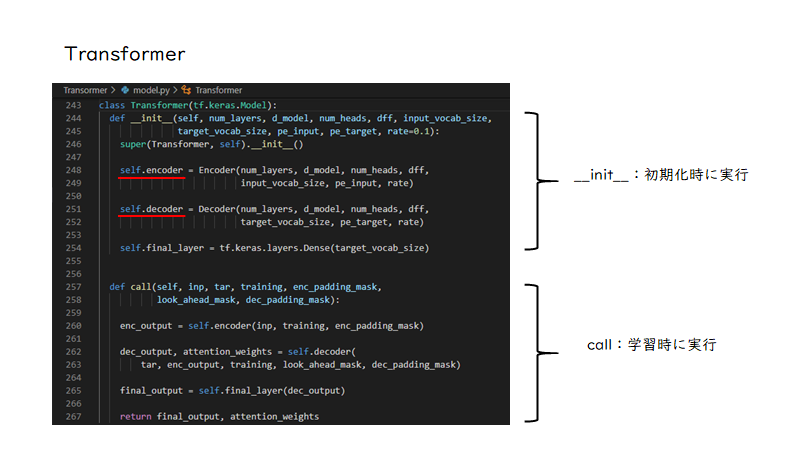
まず、Transformerクラスを見てみましょう。Transformerクラスは学習の直前に呼び出す一番表層のクラスになります。クラス内にはinit関数とcall関数があり、initはモデルを初期化した時に走る処理でモデルの要素を定義しています。また、callは学習を実行したときに走る処理が書かれており、モデルに入力したデータをどのようにデータを処理するのかを記述しています。
1 2 3 4 5 6 7 8 9 10 11 12 13 14 15 16 17 18 19 20 21 22 23 24 25 26 27 |
class Transformer(tf.keras.Model): # モデル初期化時に実行 def __init__(self, num_layers, d_model, num_heads, dff, input_vocab_size, target_vocab_size, pe_input, pe_target, rate=0.1): super(Transformer, self).__init__() self.encoder = Encoder(num_layers, d_model, num_heads, dff, input_vocab_size, pe_input, rate) self.decoder = Decoder(num_layers, d_model, num_heads, dff, target_vocab_size, pe_target, rate) self.final_layer = tf.keras.layers.Dense(target_vocab_size) # 学習時に実行 def call(self, inp, tar, training, enc_padding_mask, look_ahead_mask, dec_padding_mask): enc_output = self.encoder(inp, training, enc_padding_mask) dec_output, attention_weights = self.decoder( tar, enc_output, training, look_ahead_mask, dec_padding_mask) final_output = self.final_layer(dec_output) return final_output, attention_weights |
それを理解した上でinit関数を見てみますと、initにはEncoder, Decoderが定義されているのがわかります。そして、call関数ではencoderにinput、これは翻訳元の文が入力され、さらにdecoderにはencoderからの出力とtarget、翻訳後の文が入力されているのがわかります。
Encoder
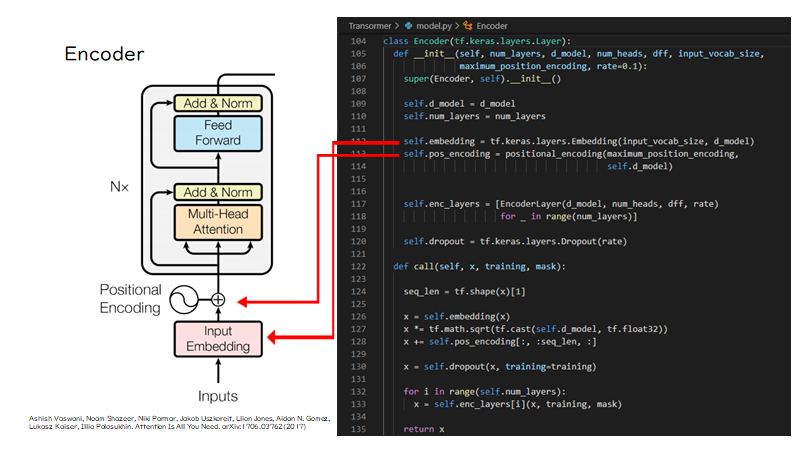
次にEncoderクラスを見てみましょう。まずinit関数ですが、左図のように、Encoderクラス内ではInput EmbeddingやPositional Encodingが定義されています。
そして、call関数では、左図のようにembeddingで分散表現が作成された後、位置情報が加算されているのがわかります。
1 2 3 4 5 6 7 8 9 10 11 12 13 14 15 16 17 18 19 20 21 22 23 24 25 26 27 28 29 30 |
class Encoder(tf.keras.layers.Layer): def __init__(self, num_layers, d_model, num_heads, dff, input_vocab_size, maximum_position_encoding, rate=0.1): super(Encoder, self).__init__() self.d_model = d_model self.num_layers = num_layers self.embedding = tf.keras.layers.Embedding(input_vocab_size, d_model) self.pos_encoding = positional_encoding(maximum_position_encoding, self.d_model) self.enc_layers = [EncoderLayer(d_model, num_heads, dff, rate) for _ in range(num_layers)] self.dropout = tf.keras.layers.Dropout(rate) def call(self, x, training, mask): seq_len = tf.shape(x)[1] x = self.embedding(x) x *= tf.math.sqrt(tf.cast(self.d_model, tf.float32)) x += self.pos_encoding[:, :seq_len, :] x = self.dropout(x, training=training) for i in range(self.num_layers): x = self.enc_layers[i](x, training, mask) return x |
Positional Encoding
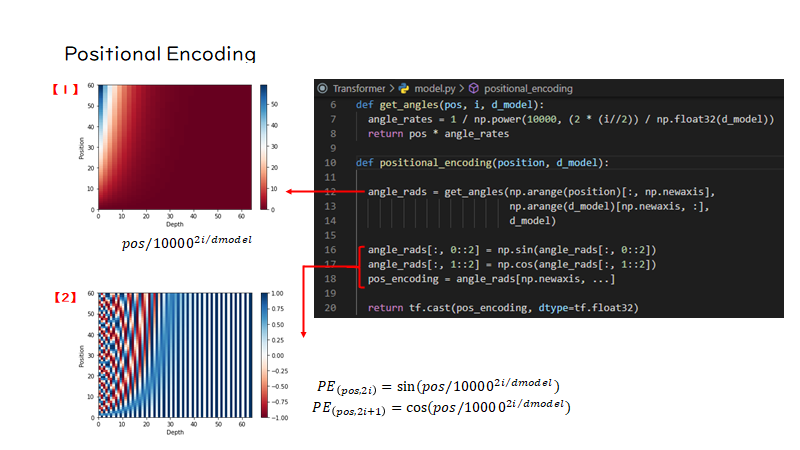
次はPositional Encoding関数の中身を見てみましょう。入力はpositionとd_modelで、positionはシーケンスの最大長、d_modelは分散表現の次元数です。
まずget_angles関数で、poistion×depthのベクトルを作成すると図【1】のようになります。図は縦軸が文章中の位置を表し、横軸がベクトルを表しています。赤いところはほとんど0に近い値で、文の先頭要素ほど、0に近い値で構成されています。
図【2】は、偶数の位置にある要素にsin関数を適用し、奇数位置にある要素にcos関数を適用したものになります。これを見ると、文章中の各要素のベクトルが各位置ごとに特徴づけられているのがわかります。こうして作成された位置情報が文ベクトルに加算されます。
1 2 3 4 5 6 7 8 9 10 11 12 13 14 15 |
def get_angles(pos, i, d_model): angle_rates = 1 / np.power(10000, (2 * (i//2)) / np.float32(d_model)) return pos * angle_rates def positional_encoding(position, d_model): angle_rads = get_angles(np.arange(position)[:, np.newaxis], np.arange(d_model)[np.newaxis, :], d_model) angle_rads[:, 0::2] = np.sin(angle_rads[:, 0::2]) angle_rads[:, 1::2] = np.cos(angle_rads[:, 1::2]) pos_encoding = angle_rads[np.newaxis, ...] return tf.cast(pos_encoding, dtype=tf.float32) |
EncoderLayer
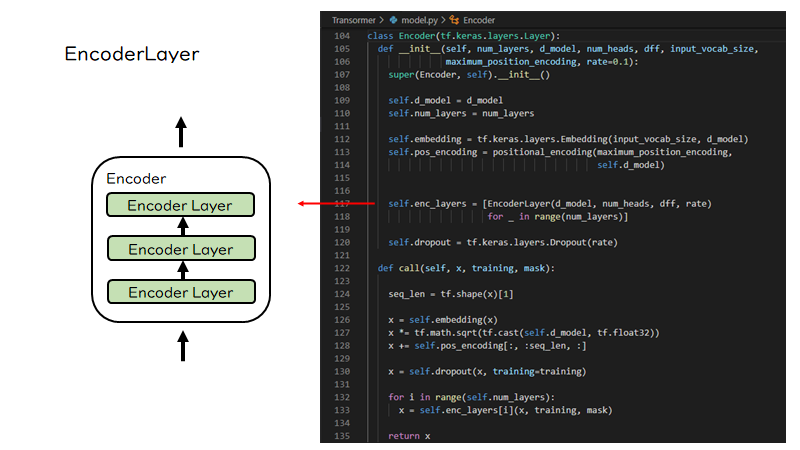
続いてEncoderLayerですが、EncoderLayerは左図のように複数積み重なっています。右のコードを見るとlist内包表記でEncoderLayerクラスがnum_layersの数だけ作られているのがわかります。そして、call関数を見ると、先ほど位置情報が加算された分散表現はdropoutレイヤーを通過した後、このEncoderLayerに入力されていきます。
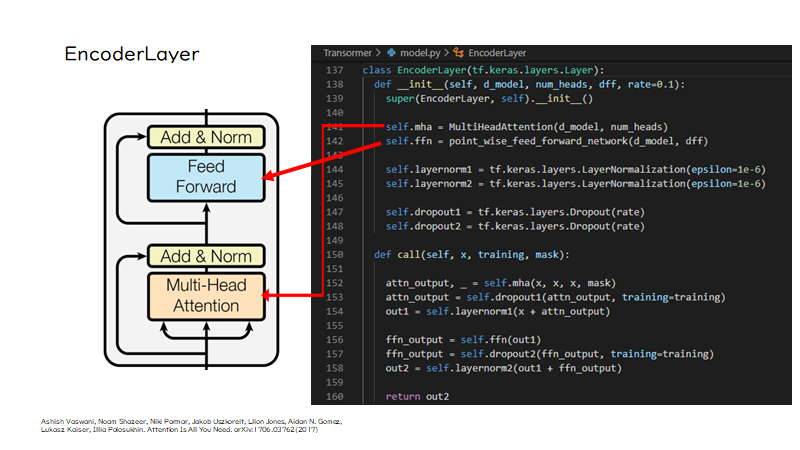
では、そのEncoderLayerの中身はどうなっているかというと、AttentionとFeed Forward Networkが定義されています。そして、call関数を見ると左図の黒い矢印ように、データが処理されているのがわかります。
ここで、mhaというのはMulti Head Attentionクラスになりますが、これに翻訳元の文ベクトルxが代入されているのがわかります。なぜxが3つあるかというと、以前説明したように、Self Attetnionの計算で、自己のベクトルを掛け合わせているからです。
1 2 3 4 5 6 7 8 9 10 11 12 13 14 15 16 17 18 19 20 21 22 23 24 |
class EncoderLayer(tf.keras.layers.Layer): def __init__(self, d_model, num_heads, dff, rate=0.1): super(EncoderLayer, self).__init__() self.mha = MultiHeadAttention(d_model, num_heads) self.ffn = point_wise_feed_forward_network(d_model, dff) self.layernorm1 = tf.keras.layers.LayerNormalization(epsilon=1e-6) self.layernorm2 = tf.keras.layers.LayerNormalization(epsilon=1e-6) self.dropout1 = tf.keras.layers.Dropout(rate) self.dropout2 = tf.keras.layers.Dropout(rate) def call(self, x, training, mask): attn_output, _ = self.mha(x, x, x, mask) attn_output = self.dropout1(attn_output, training=training) out1 = self.layernorm1(x + attn_output) ffn_output = self.ffn(out1) ffn_output = self.dropout2(ffn_output, training=training) out2 = self.layernorm2(out1 + ffn_output) return out2 |
Multi-Head Attention
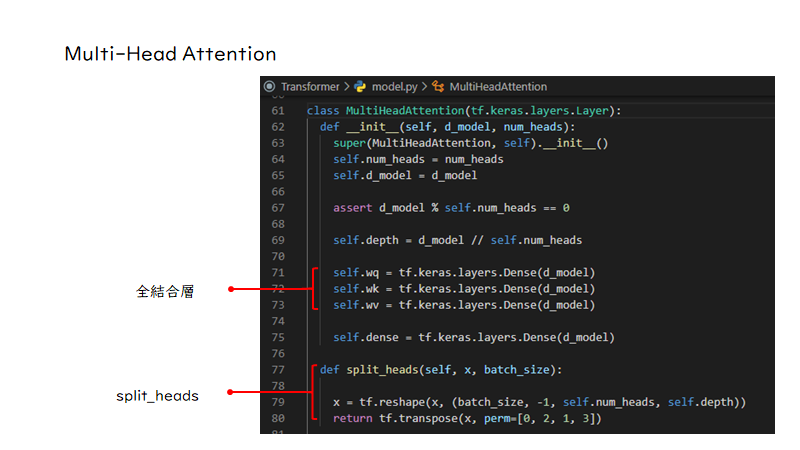
次にMulti Head Attentionですが、これはクラスのinit関数だけ表示しています。init関数を見てみると、全結合層とsplit_heads関数も定義されており、Multi Head Attentionの主な構成要素はこの二つであることがわかります。
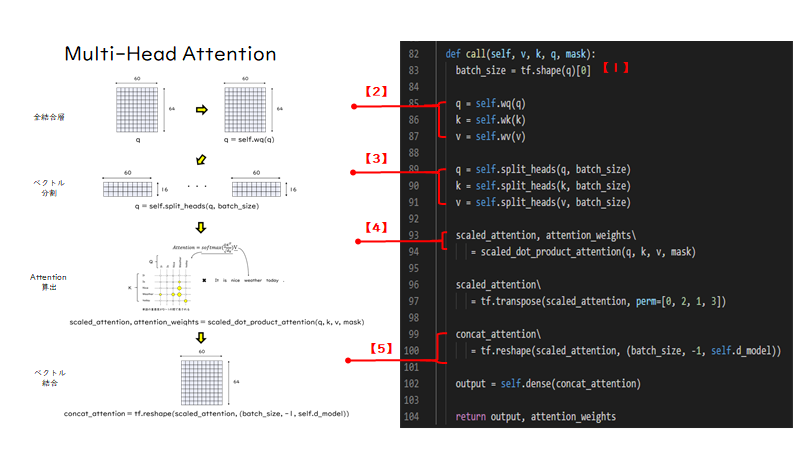
次に、call関数を見てみましょう。右図がソースコード、左図はソースコードのcall関数での処理を図示したものになります。ここでは、文ベクトルqを例にcall関数でどのように処理されているのかを説明します。まず、batch_size = tf.shape(q)[0]とありますが、これはバッチサイズを取得しています。【1】
そして、q = self.wq(q)は、init関数で定義した全結合層に文ベクトルを入力しています。【2】
イメージとしては、左図のようになります。今回は出力サイズが分散表現の次元と同じなので、形状は変化していません。
続いて、その出力がsplit_heads関数に入力されいます【3】、この時、先ほど取得したバッチサイズも入力されています。split_heads関数では、左図のように文ベクトルを分割しています。分割された後は、scaled_dot_product_attention関数に入力され、ここで、Attentionが計算されます。【4】
Attentionが計算されると文ベクトルとAttentionの重みを出力しますが、文ベクトルは再び結合されます。【5】
1 2 3 4 5 6 7 8 9 10 11 12 13 14 15 16 17 18 19 20 21 22 23 24 25 26 27 28 29 30 31 32 33 34 35 36 37 38 39 40 41 |
class MultiHeadAttention(tf.keras.layers.Layer): def __init__(self, d_model, num_heads): super(MultiHeadAttention, self).__init__() self.num_heads = num_heads self.d_model = d_model assert d_model % self.num_heads == 0 self.depth = d_model // self.num_heads self.wq = tf.keras.layers.Dense(d_model) self.wk = tf.keras.layers.Dense(d_model) self.wv = tf.keras.layers.Dense(d_model) self.dense = tf.keras.layers.Dense(d_model) def split_heads(self, x, batch_size): x = tf.reshape(x, (batch_size, -1, self.num_heads, self.depth)) return tf.transpose(x, perm=[0, 2, 1, 3]) def call(self, v, k, q, mask): batch_size = tf.shape(q)[0] q = self.wq(q) k = self.wk(k) v = self.wv(v) q = self.split_heads(q, batch_size) k = self.split_heads(k, batch_size) v = self.split_heads(v, batch_size) scaled_attention, attention_weights = scaled_dot_product_attention(q, k, v, mask) scaled_attention = tf.transpose(scaled_attention, perm=[0, 2, 1, 3]) concat_attention = tf.reshape(scaled_attention, (batch_size, -1, self.d_model)) output = self.dense(concat_attention) return output, attention_weights |
Scaled Dot-Product Attention
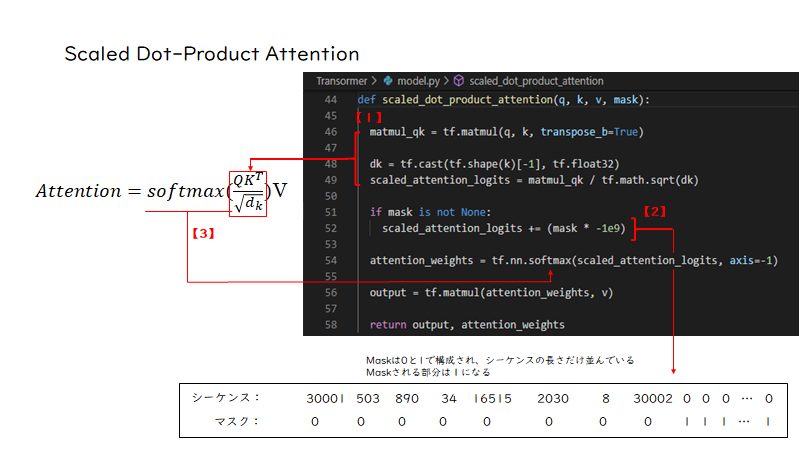
scaled_dot_product_attention関数ですが、引数にq,k,vとmaskを取ります。
やっている処理は左式の通りですが、まず上の3行でsoftmaxのカッコ内の計算をしています。【1】
そして、maskがNone出ない場合は、上の3行で計算した結果にmaskが加算されます。【2】ここでmaskは下に記載してあるように、シーケンスと同じ長さの0と1の要素から構成される配列になります。maskされている部分、つまりAttentionの計算時に無視したい部分には1が入っています。この時0埋めしたシーケンスの場合、0は無視したいということがあります。ここで-10の9乗の値をかけていますが、1が入っているとその要素Attentionは大きなマイナスの値になります。つまりその位置の単語の重要度は小さくなるというわけです。このマスクは、他にもDecoderのMasked Multi Head Attentionでも使われています。
1 2 3 4 5 6 7 8 9 10 11 12 13 14 15 |
def scaled_dot_product_attention(q, k, v, mask): matmul_qk = tf.matmul(q, k, transpose_b=True) dk = tf.cast(tf.shape(k)[-1], tf.float32) scaled_attention_logits = matmul_qk / tf.math.sqrt(dk) if mask is not None: scaled_attention_logits += (mask * -1e9) attention_weights = tf.nn.softmax(scaled_attention_logits, axis=-1) output = tf.matmul(attention_weights, v) return output, attention_weights |
おわりに
今回はソースコードと比較しながら、Transformerのモデルアーキテクチャや学習の過程について解説しました。解説内容はEncoderに沿ったものでしたが、Decoderも同じように追っていけば理解できますので、ご自身で確認してみてください。また、理解しがたい部分に関しては、コードを切り出してきて具体的な数値で実行してみると何をやっているかつかめるかと思います。
(K. K)